
How to analyze a Codebase using ChatGPT and o3
AI is rapidly evolving. Recent models like Google Gemini 2.5 support context windows up to 1 million tokens—meaning we can potentially fit an entire codebase, documentation, and instructions into a single prompt.
That’s incredibly powerful... but also costly. Running queries on large contexts can quickly burn through API credits or subscription limits.
What if there’s a more efficient way to get the same result, without maxing out your plan?
Let’s dive into a practical approach using ChatGPT o3 and an open-source tool called Repomix.
Pack the Code
Before throwing a codebase at an AI, we need to structure it into a compact, analyzable format.
The original tool in this space is Repo Prompt, which lets you open a project, select files, and craft your prompt. It works, but, it's a bit clunky. You need to install the app, select files manually, and structure everything by hand.
Here’s a cleaner alternative: Repomix. It's a Node.js package that packs your codebase into a compressed XML format using Tree-sitter under the hood, the same parser used in tools like Aider AI and some modern IDEs.
Run it from the terminal with one command:
npx repomix --include "src/**/*.ts,**/*.md" --compress
This creates a repomix-output.xml
file, a compact snapshot of your project—ready to feed into an AI.
A Real Use Case
Let’s walk through a real-world scenario. I was digging into the TypeScript Language Server and wanted to understand how the textDocument/diagnostic
endpoint was implemented—specifically from the LSP 3.17 spec.
Although Repomix supports remote repositories, I prefer cloning locally to freely explore the code:
# Clone the repo
git clone https://github.com/typescript-language-server/typescript-language-server.git
cd typescript-language-server
# Pack the relevant files
npx repomix --include "src/**/*.ts,**/*.md" --compress
That’s it. We now have a compact, context-rich file for the AI to analyze.
Let ChatGPT o3 Do the Heavy Lifting
Next step: analysis.
ChatGPT’s o3 model is a game-changer. It combines deep reasoning, web search, and code awareness, all while staying concise and clear.
At the time of writing, the $20/month ChatGPT Plus plan gives access to o3, but it still comes with a limited context window. So instead of pasting the entire XML, we just upload the repomix-output.xml
file directly into the chat.
Here’s the prompt I used:
Given this repo, how does the workspace/diagnostic or textDocument/diagnostic work?
That’s it. No need for fancy instructions or long-winded prompts.
The o3 model responded by generating Python code to parse the XML and even performed web searches to cross-reference the LSP spec and validate the diagnostic flow in the code.
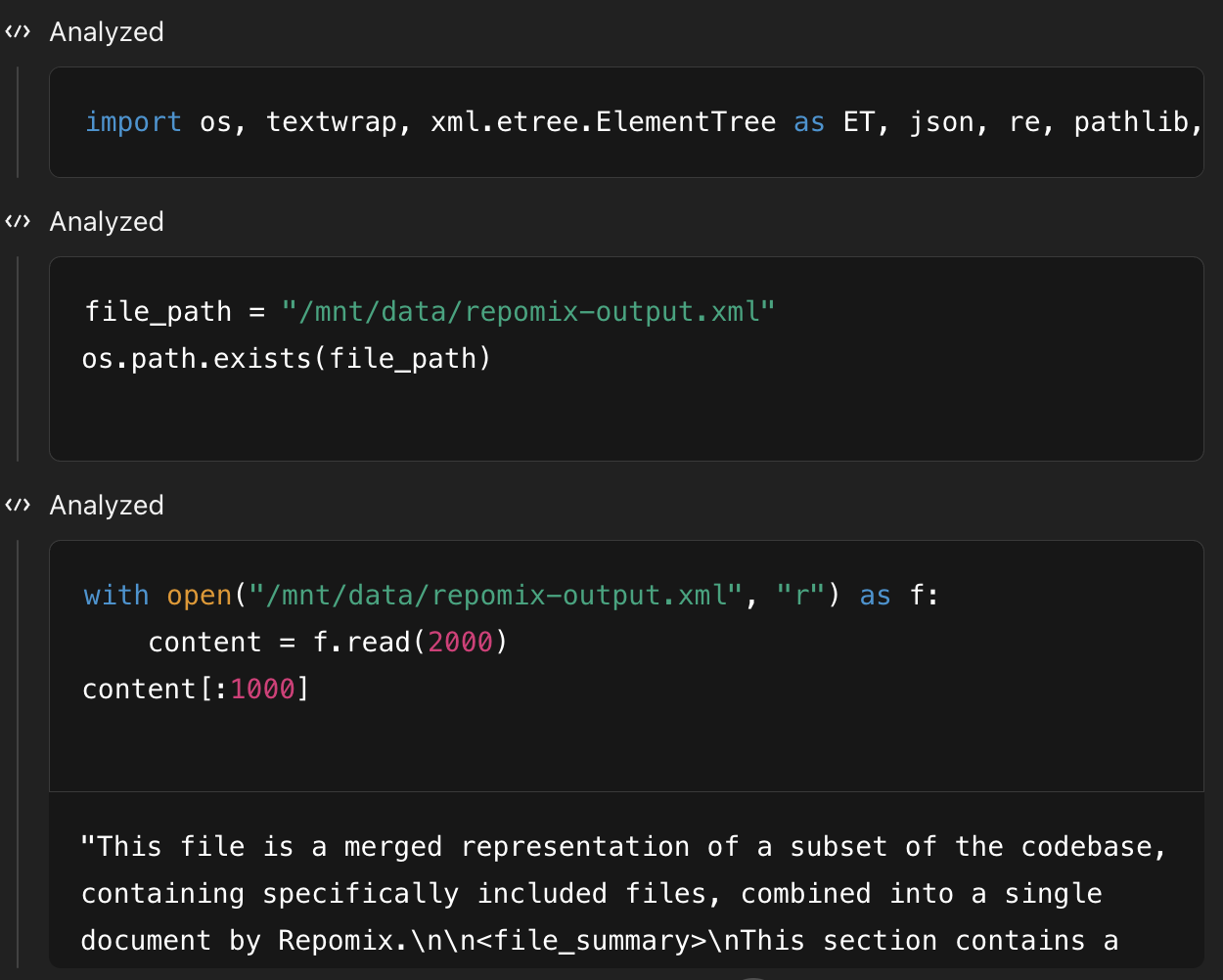
🧠 Cool detail: It refined the Python code over time, improved XML parsing, and used search terms that led it straight to the implementation details.
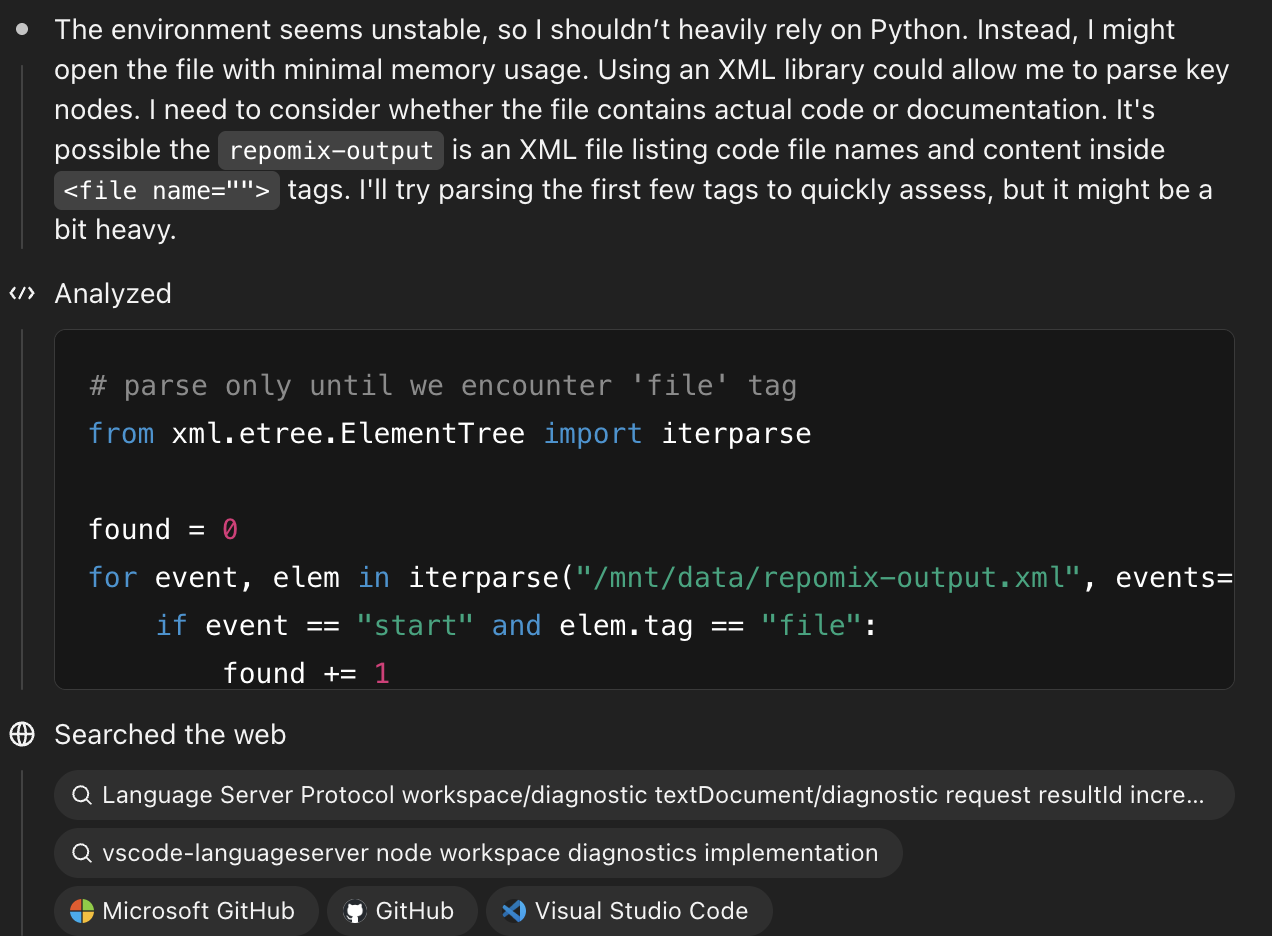
Results
By the end of the chat, I had a complete understanding of:
- How diagnostics are enabled and configured in the project
- Where and how
workspace/diagnostic
andtextDocument/diagnostic
are handled - What functions were responsible for triggering and formatting diagnostics
o3 even returned a TL;DR summary and information table breaking down the relevant files, classes, and responsibilities. Super useful when you're onboarding or debugging.
You can read the full chat here: ChatGPT shared chat
Final Thoughts
I was genuinely surprised by how far you can go with just a ChatGPT Plus subscription and a simple repomix file. o3 didn’t need detailed instructions—it just figured it out from the compressed codebase and used its reasoning + search to provide insights.
It’s exciting to see AI move from simple Q&A toward true code reasoning and understanding. You don’t need advanced prompting anymore, just a simple sentence and the right tools.